Publications
For news about publications, follow us on X: 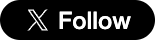
Click on any author names or tags to filter publications.
All topic tags:
surveydeep-rlmulti-agent-rlagent-modellingad-hoc-teamworkautonomous-drivinggoal-recognitionexplainable-aicausalgeneralisationsecurityemergent-communicationiterated-learningintrinsic-rewardsimulatorstate-estimationdeep-learningtransfer-learning
Selected tags (click to remove):
Christopher-G.-Lucas
2024
Samuel Garcin, James Doran, Shangmin Guo, Christopher G. Lucas, Stefano V. Albrecht
DRED: Zero-Shot Transfer in Reinforcement Learning via Data-Regularised Environment Design
International Conference on Machine Learning, 2024
Abstract | BibTex | arXiv
ICMLdeep-rl
Abstract:
Autonomous agents trained using deep reinforcement learning (RL) often lack the ability to successfully generalise to new environments, even when they share characteristics with the environments they have encountered during training. In this work, we investigate how the sampling of individual environment instances, or levels, affects the zero-shot generalisation (ZSG) ability of RL agents. We discover that, for deep actor-critic architectures sharing their base layers, prioritising levels according to their value loss minimises the mutual information between the agent's internal representation and the set of training levels in the generated training data. This provides a novel theoretical justification for the implicit regularisation achieved by certain adaptive sampling strategies. We then turn our attention to unsupervised environment design (UED) methods, which have more control over the data generation mechanism. We find that existing UED methods can significantly shift the training distribution, which translates to low ZSG performance. To prevent both overfitting and distributional shift, we introduce data-regularised environment design (DRED). DRED generates levels using a generative model trained over an initial set of level parameters, reducing distributional shift, and achieves significant improvements in ZSG over adaptive level sampling strategies and UED methods.
@inproceedings{garcin2024dred,
title={{DRED}: Zero-Shot Transfer in Reinforcement Learning via Data-Regularised Environment Design},
author={Samuel Garcin and James Doran and Shangmin Guo and Christopher G. Lucas and Stefano V. Albrecht},
year={2024},
booktitle={International Conference on Machine Learning (ICML)}
}
Balint Gyevnar, Cheng Wang, Christopher G. Lucas, Shay B. Cohen, Stefano V. Albrecht
Causal Explanations for Sequential Decision-Making in Multi-Agent Systems
International Conference on Autonomous Agents and Multi-Agent Systems, 2024
Abstract | BibTex | arXiv | Code | Dataset
AAMASexplainable-aiautonomous-drivingcausal
Abstract:
We present CEMA: Causal Explanations in Multi-Agent systems; a framework for creating causal natural language explanations of an agent's decisions in dynamic sequential multi-agent systems to build more trustworthy autonomous agents. Unlike prior work that assumes a fixed causal structure, CEMA only requires a probabilistic model for forward-simulating the state of the system. Using such a model, CEMA simulates counterfactual worlds that identify the salient causes behind the agent's decisions. We evaluate CEMA on the task of motion planning for autonomous driving and test it in diverse simulated scenarios. We show that CEMA correctly and robustly identifies the causes behind the agent's decisions, even when a large number of other agents is present, and show via a user study that CEMA's explanations have a positive effect on participants' trust in autonomous vehicles and are rated as high as high-quality baseline explanations elicited from other participants.
@inproceedings{gyevnar2024cema,
title={Causal Explanations for Sequential Decision-Making in Multi-Agent Systems},
author={Balint Gyevnar and Cheng Wang and Christopher G. Lucas and Shay B. Cohen and Stefano V. Albrecht},
booktitle = {Proceedings of the 23rd International Conference on Autonomous Agents and Multiagent Systems},
year={2024}
}
2023
Samuel Garcin, James Doran, Shangmin Guo, Christopher G. Lucas, Stefano V. Albrecht
How the level sampling process impacts zero-shot generalisation in deep reinforcement learning
NeurIPS Workshop on Agent Learning in Open-Endedness, 2023
Abstract | BibTex | arXiv
NeurIPSdeep-rl
Abstract:
A key limitation preventing the wider adoption of autonomous agents trained via deep reinforcement learning (RL) is their limited ability to generalise to new environments, even when these share similar characteristics with environments encountered during training. In this work, we investigate how a non-uniform sampling strategy of individual environment instances, or levels, affects the zero-shot generalisation (ZSG) ability of RL agents, considering two failure modes: overfitting and over-generalisation. As a first step, we measure the mutual information (MI) between the agent's internal representation and the set of training levels, which we find to be well-correlated to instance overfitting. In contrast to uniform sampling, adaptive sampling strategies prioritising levels based on their value loss are more effective at maintaining lower MI, which provides a novel theoretical justification for this class of techniques. We then turn our attention to unsupervised environment design (UED) methods, which adaptively generate new training levels and minimise MI more effectively than methods sampling from a fixed set. However, we find UED methods significantly shift the training distribution, resulting in over-generalisation and worse ZSG performance over the distribution of interest. To prevent both instance overfitting and over-generalisation, we introduce self-supervised environment design (SSED). SSED generates levels using a variational autoencoder, effectively reducing MI while minimising the shift with the distribution of interest, and leads to statistically significant improvements in ZSG over fixed-set level sampling strategies and UED methods.
@inproceedings{garcin2023level,
title={How the level sampling process impacts zero-shot generalisation in deep reinforcement learning},
author={Samuel Garcin and James Doran and Shangmin Guo and Christopher G. Lucas and Stefano V. Albrecht},
booktitle={NeurIPS Workshop on Agent Learning in Open-Endedness},
year={2023}
}
Balint Gyevnar, Cheng Wang, Christopher G. Lucas, Shay B. Cohen, Stefano V. Albrecht
Causal Social Explanations for Stochastic Sequential Multi-Agent Decision-Making
AAMAS Workshop on Explainable and Transparent AI and Multi-Agent Systems, 2023
Abstract | BibTex | arXiv | Code
AAMASautonomous-drivingexplainable-aicausal
Abstract:
We present a novel framework to generate causal explanations for the decisions of agents in stochastic sequential multi-agent environments. Explanations are given via natural language conversations answering a wide range of user queries and requiring associative, interventionist, or counterfactual causal reasoning. Instead of assuming any specific causal graph, our method relies on a generative model of interactions to simulate counterfactual worlds which are used to identify the salient causes behind decisions. We implement our method for motion planning for autonomous driving and test it in simulated scenarios with coupled interactions. Our method correctly identifies and ranks the relevant causes and delivers concise explanations to the users' queries.
@inproceedings{gyevnar2023causal,
title={Causal Social Explanations for Stochastic Sequential Multi-Agent Decision-Making},
author={Balint Gyevnar and Cheng Wang and Christopher G. Lucas and Shay B. Cohen and Stefano V. Albrecht},
booktitle={5th International Workshop on EXplainable and TRAnsparent AI and Multi-Agent Systems},
year={2023}
}
Samuel Garcin, James Doran, Shangmin Guo, Christopher G. Lucas, Stefano V. Albrecht
How the level sampling process impacts zero-shot generalisation in deep reinforcement learning
arXiv:2310.03494, 2023
Abstract | BibTex | arXiv
deep-rl
Abstract:
A key limitation preventing the wider adoption of autonomous agents trained via deep reinforcement learning (RL) is their limited ability to generalise to new environments, even when these share similar characteristics with environments encountered during training. In this work, we investigate how a non-uniform sampling strategy of individual environment instances, or levels, affects the zero-shot generalisation (ZSG) ability of RL agents, considering two failure modes: overfitting and over-generalisation. As a first step, we measure the mutual information (MI) between the agent's internal representation and the set of training levels, which we find to be well-correlated to instance overfitting. In contrast to uniform sampling, adaptive sampling strategies prioritising levels based on their value loss are more effective at maintaining lower MI, which provides a novel theoretical justification for this class of techniques. We then turn our attention to unsupervised environment design (UED) methods, which adaptively generate new training levels and minimise MI more effectively than methods sampling from a fixed set. However, we find UED methods significantly shift the training distribution, resulting in over-generalisation and worse ZSG performance over the distribution of interest. To prevent both instance overfitting and over-generalisation, we introduce self-supervised environment design (SSED). SSED generates levels using a variational autoencoder, effectively reducing MI while minimising the shift with the distribution of interest, and leads to statistically significant improvements in ZSG over fixed-set level sampling strategies and UED methods.
@misc{garcin2023level,
title={How the level sampling process impacts zero-shot generalisation in deep reinforcement learning},
author={Samuel Garcin and James Doran and Shangmin Guo and Christopher G. Lucas and Stefano V. Albrecht},
year={2023},
eprint={2310.03494},
archivePrefix={arXiv},
primaryClass={cs.LG}
}
2022
Balint Gyevnar, Massimiliano Tamborski, Cheng Wang, Christopher G. Lucas, Shay B. Cohen, Stefano V. Albrecht
A Human-Centric Method for Generating Causal Explanations in Natural Language for Autonomous Vehicle Motion Planning
IJCAI Workshop on Artificial Intelligence for Autonomous Driving, 2022
Abstract | BibTex | arXiv | Code
IJCAIautonomous-drivingexplainable-aicausal
Abstract:
Inscrutable AI systems are difficult to trust, especially if they operate in safety-critical settings like autonomous driving. Therefore, there is a need to build transparent and queryable systems to increase trust levels. We propose a transparent, human-centric explanation generation method for autonomous vehicle motion planning and prediction based on an existing white-box system called IGP2. Our method integrates Bayesian networks with context-free generative rules and can give causal natural language explanations for the high-level driving behaviour of autonomous vehicles. Preliminary testing on simulated scenarios shows that our method captures the causes behind the actions of autonomous vehicles and generates intelligible explanations with varying complexity.
@inproceedings{gyevnar2022humancentric,
title={A Human-Centric Method for Generating Causal Explanations in Natural Language for Autonomous Vehicle Motion Planning},
author={Balint Gyevnar and Massimiliano Tamborski and Cheng Wang and Christopher G. Lucas and Shay B. Cohen and Stefano V. Albrecht},
booktitle={IJCAI Workshop on Artificial Intelligence for Autonomous Driving},
year={2022}
}