Publications
For news about publications, follow us on X: 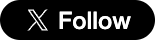
Click on any author names or tags to filter publications.
All topic tags:
surveydeep-rlmulti-agent-rlagent-modellingad-hoc-teamworkautonomous-drivinggoal-recognitionexplainable-aicausalgeneralisationsecurityemergent-communicationiterated-learningintrinsic-rewardsimulatorstate-estimationdeep-learningtransfer-learning
Selected tags (click to remove):
David-Mguni
2025
Lukas Schäfer, Oliver Slumbers, Stephen Mcaleer, Yali Du, Stefano V. Albrecht, David Mguni
Ensemble Value Functions for Efficient Exploration in Multi-Agent Reinforcement Learning
International Conference on Autonomous Agents and Multi-Agent Systems, 2025
Abstract | BibTex | arXiv
AAMASmulti-agent-rl
Abstract:
Multi-agent reinforcement learning (MARL) requires agents to explore within a vast joint action space to find joint actions that lead to coordination. Existing value-based MARL algorithms commonly rely on random exploration, such as ϵ-greedy, to explore the environment which is not systematic and inefficient at identifying effective actions in multi-agent problems. Additionally, the concurrent training of the policies of multiple agents during training can render the optimisation non-stationary. This can lead to unstable value estimates, highly variant gradients, and ultimately hinder coordination between agents. To address these challenges, we propose ensemble value functions for multi-agent exploration (EMAX). EMAX is a framework to seamlessly extend value-based MARL algorithms. EMAX leverages an ensemble of value functions for each agent to guide their exploration, reduce the variance of their optimisation, and makes their policies more robust to miscoordination. EMAX achieves these benefits by (1) systematically guiding the exploration of agents with a UCB policy towards parts of the environment that require multiple agents to coordinate. (2) EMAX computes average value estimates across the ensemble as target values to reduce the variance of gradients and make optimisation more stable. (3) During evaluation, EMAX selects actions following a majority vote across the ensemble to reduce the likelihood of miscoordination. We first instantiate independent DQN with EMAX and evaluate it in 11 general-sum tasks with sparse rewards. We show that EMAX improves final evaluation returns by 185% across all tasks. We then evaluate EMAX on top of IDQN, VDN and QMIX in 21 common-reward tasks, and show that EMAX improves sample efficiency and final evaluation returns across all tasks over all three vanilla algorithms by 60%, 47%, and 538%, respectively.
@inproceedings{schafer2025emax,
title = {Ensemble Value Functions for Efficient Exploration in Multi-Agent Reinforcement Learning},
author = {Lukas Sch\"afer and Oliver Slumbers and Stephen Mcaleer and Yali Du and Stefano V. Albrecht and David Mguni},
booktitle = {International Conference on Autonomous Agents and Multiagent Systems},
year = {2025}
}
2023
Lukas Schäfer, Oliver Slumbers, Stephen McAleer, Yali Du, Stefano V. Albrecht, David Mguni
Ensemble Value Functions for Efficient Exploration in Multi-Agent Reinforcement Learning
AAMAS Workshop on Adaptive and Learning Agents, 2023
Abstract | BibTex | arXiv
AAMASmulti-agent-rldeep-rl
Abstract:
Cooperative multi-agent reinforcement learning (MARL) requires agents to explore to learn to cooperate. Existing value-based MARL algorithms commonly rely on random exploration, such as ϵ-greedy, which is inefficient in discovering multi-agent cooperation. Additionally, the environment in MARL appears non-stationary to any individual agent due to the simultaneous training of other agents, leading to highly variant and thus unstable optimisation signals. In this work, we propose ensemble value functions for multi-agent exploration (EMAX), a general framework to extend any value-based MARL algorithm. EMAX trains ensembles of value functions for each agent to address the key challenges of exploration and non-stationarity: (1) The uncertainty of value estimates across the ensemble is used in a UCB policy to guide the exploration of agents to parts of the environment which require cooperation. (2) Average value estimates across the ensemble serve as target values. These targets exhibit lower variance compared to commonly applied target networks and we show that they lead to more stable gradients during the optimisation. We instantiate three value-based MARL algorithms with EMAX, independent DQN, VDN and QMIX, and evaluate them in 21 tasks across four environments. Using ensembles of five value functions, EMAX improves sample efficiency and final evaluation returns of these algorithms by 53%, 36%, and 498%, respectively, averaged all 21 tasks.
@inproceedings{schaefer2023emax,
title={Ensemble Value Functions for Efficient Exploration in Multi-Agent Reinforcement Learning},
author={Lukas Schäfer and Oliver Slumbers and Stephen McAleer and Yali Du and Stefano V. Albrecht and David Mguni},
year={2023},
booktitle={AAMAS Workshop on Adaptive and Learning Agents (ALA)},
}