Publications
For news about publications, follow us on X: 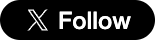
Click on any author names or tags to filter publications.
All topic tags:
surveydeep-rlmulti-agent-rlagent-modellingad-hoc-teamworkautonomous-drivinggoal-recognitionexplainable-aicausalgeneralisationsecurityemergent-communicationiterated-learningintrinsic-rewardsimulatorstate-estimationdeep-learningtransfer-learning
Selected tags (click to remove):
Elliot-FosongIJCAI
2022
Arrasy Rahman, Elliot Fosong, Ignacio Carlucho, Stefano V. Albrecht
Towards Robust Ad Hoc Teamwork Agents By Creating Diverse Training Teammates
IJCAI Workshop on Ad Hoc Teamwork, 2022
Abstract | BibTex | arXiv | Code
IJCAIad-hoc-teamworkmulti-agent-rl
Abstract:
Ad hoc teamwork (AHT) is the problem of creating an agent that must collaborate with previously unseen teammates without prior coordination. Many existing AHT methods can be categorised as type-based methods, which require a set of predefined teammates for training. Designing teammate types for training is a challenging issue that determines the generalisation performance of agents when dealing with teammate types unseen during training. In this work, we propose a method to discover diverse teammate types based on maximising best response diversity metrics. We show that our proposed approach yields teammate types that require a wider range of best responses from the learner during collaboration, which potentially improves the robustness of a learner's performance in AHT compared to alternative methods.
@inproceedings{rahman2022towards,
title={Towards Robust Ad Hoc Teamwork Agents By Creating Diverse Training Teammates},
author={Arrasy Rahman and Elliot Fosong and Ignacio Carlucho and Stefano V. Albrecht},
booktitle={IJCAI Workshop on Ad Hoc Teamwork},
year={2022}
}
Elliot Fosong, Arrasy Rahman, Ignacio Carlucho, Stefano V. Albrecht
Few-Shot Teamwork
IJCAI Workshop on Ad Hoc Teamwork, 2022
Abstract | BibTex | arXiv
IJCAIad-hoc-teamworkmulti-agent-rl
Abstract:
We propose the novel few-shot teamwork (FST) problem, where skilled agents trained in a team to complete one task are combined with skilled agents from different tasks, and together must learn to adapt to an unseen but related task. We discuss how the FST problem can be seen as addressing two separate problems: one of reducing the experience required to train a team of agents to complete a complex task; and one of collaborating with unfamiliar teammates to complete a new task. Progress towards solving FST could lead to progress in both multi-agent reinforcement learning and ad hoc teamwork.
@inproceedings{fosong2022fewshot,
title={Few-Shot Teamwork},
author={Elliot Fosong and Arrasy Rahman and Ignacio Carlucho and Stefano V. Albrecht},
booktitle={IJCAI Workshop on Ad Hoc Teamwork},
year={2022}
}
Ignacio Carlucho, Arrasy Rahman, William Ard, Elliot Fosong, Corina Barbalata, Stefano V. Albrecht
Cooperative Marine Operations Via Ad Hoc Teams
IJCAI Workshop on Ad Hoc Teamwork, 2022
Abstract | BibTex | arXiv
IJCAIad-hoc-teamworkmulti-agent-rl
Abstract:
While research in ad hoc teamwork has great potential for solving real-world robotic applications, most developments so far have been focusing on environments with simple dynamics. In this article, we discuss how the problem of ad hoc teamwork can be of special interest for marine robotics and how it can aid marine operations. Particularly, we present a set of challenges that need to be addressed for achieving ad hoc teamwork in underwater environments and we discuss possible solutions based on current state-of-the-art developments in the ad hoc teamwork literature.
@inproceedings{Carlucho2022UnderwaterAHT,
title={Cooperative Marine Operations Via Ad Hoc Teams},
author={Ignacio Carlucho, Arrasy Rahman, William Ard, Elliot Fosong, Corina Barbalata, Stefano V. Albrecht},
booktitle={IJCAI Workshop on Ad Hoc Teamwork},
year={2022}
}