Publications
For news about publications, follow us on X: 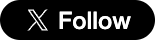
Click on any author names or tags to filter publications.
All topic tags:
surveydeep-rlmulti-agent-rlagent-modellingad-hoc-teamworkautonomous-drivinggoal-recognitionexplainable-aicausalgeneralisationsecurityemergent-communicationiterated-learningintrinsic-rewardsimulatorstate-estimationdeep-learningtransfer-learning
Selected tags (click to remove):
ICLR
2025
Samuel Garcin, Trevor McInroe, Pablo Samuel Castro, Christopher G. Lucas, David Abel, Prakash Panangaden, Stefano V. Albrecht
Studying the Interplay Between the Actor and Critic Representations in Reinforcement Learning
International Conference on Learning Representations, 2025
Abstract | BibTex | Paper | Code
ICLRdeep-rlgeneralisation
Abstract:
Extracting relevant information from a stream of high-dimensional observations is a central challenge for deep reinforcement learning agents. Actor-critic algorithms add further complexity to this challenge, as it is often unclear whether the same information will be relevant to both the actor and the critic. To this end, we here explore the principles that underlie effective representations for an actor and for a critic. We focus our study on understanding whether an actor and a critic will benefit from a decoupled, rather than shared, representation. Our primary finding is that when decoupled, the representations for the actor and critic systematically specialise in extracting different types of information from the environment---the actor's representation tends to focus on action-relevant information, while the critic's representation specialises in encoding value and dynamics information. Finally, we demonstrate how these insights help select representation learning objectives that play into the actor's and critic's respective knowledge specialisations, and improve performance in terms of agent returns.
@inproceedings{garcin2025acrep,
title={Studying the Interplay Between the Actor and Critic Representations in Reinforcement Learning},
author={Samuel Garcin and Trevor McInroe and Pablo Samuel Castro and Christopher G. Lucas and David Abel and Prakash Panangaden and Stefano V. Albrecht},
booktitle={13th International Conference on Learning Representations},
year={2025}
}
Xu Liu, Haobo Fu, Stefano V. Albrecht, Qiang Fu, Shuai Li
Online-to-Offline RL for Agent Alignment
International Conference on Learning Representations, 2025
Abstract | BibTex | Paper | Code
ICLRdeep-learning
Abstract:
Reinforcement learning (RL) has shown remarkable success in training agents to achieve high-performing policies, particularly in domains like Game AI where simulation environments enable efficient interactions. However, despite their success in maximizing these returns, such online-trained policies often fail to align with human preferences concerning actions, styles, and values. The challenge lies in efficiently adapting these online-trained policies to align with human preferences, given the scarcity and high cost of collecting human behavior data. In this work, we formalize the problem as online-to-offline RL and propose ALIGNment of Game AI to Preferences (ALIGN-GAP), an innovative approach for the alignment of well-trained game agents to human preferences. Our method features a carefully designed reward model that encodes human preferences from limited offline data and incorporates curriculum-based preference learning to align RL agents with targeted human preferences. Experiments across diverse environments and preference types demonstrate the performance of ALIGN-GAP, achieving effective alignment with human preferences.
@inproceedings{liu2025aligngap,
title={Online-to-Offline RL for Agent Alignment},
author={Xu Liu and Haobo Fu and Stefano V. Albrecht and Qiang Fu and Shuai Li},
booktitle={13th International Conference on Learning Representations},
year={2025}
}
2024
Shangmin Guo, Yi Ren, Stefano V. Albrecht, Kenny Smith
lpNTK: Better Generalisation with Less Data via Sample Interaction During Learning
International Conference on Learning Representations, 2024
Abstract | BibTex | arXiv | Code
ICLRdeep-learning
Abstract:
Although much research has been done on proposing new models or loss functions to improve the generalisation of artificial neural networks (ANNs), less attention has been directed to the impact of the training data on generalisation. In this work, we start from approximating the interaction between samples, i.e. how learning one sample would modify the model's prediction on other samples. Through analysing the terms involved in weight updates in supervised learning, we find that labels influence the interaction between samples. Therefore, we propose the labelled pseudo Neural Tangent Kernel (lpNTK) which takes label information into consideration when measuring the interactions between samples. We first prove that lpNTK asymptotically converges to the empirical neural tangent kernel in terms of the Frobenius norm under certain assumptions. Secondly, we illustrate how lpNTK helps to understand learning phenomena identified in previous work, specifically the learning difficulty of samples and forgetting events during learning. Moreover, we also show that using lpNTK to identify and remove poisoning training samples does not hurt the generalisation performance of ANNs.
@inproceedings{guo2024lpntk,
title={Sample Relationship from Learning Dynamics Matters for Generalisation},
author={Shangmin Guo and Yi Ren and Stefano V. Albrecht and Kenny Smith},
booktitle={12th International Conference on Learning Representations},
year={2024},
url={https://openreview.net/forum?id=8Ju0VmvMCW}
}
2023
Mhairi Dunion, Trevor McInroe, Kevin Sebastian Luck, Josiah Hanna, Stefano V. Albrecht
Temporal Disentanglement of Representations for Improved Generalisation in Reinforcement Learning
International Conference on Learning Representations, 2023
Abstract | BibTex | arXiv | Code
ICLRdeep-rlgeneralisationcausal
Abstract:
Reinforcement Learning (RL) agents are often unable to generalise well to environment variations in the state space that were not observed during training. This issue is especially problematic for image-based RL, where a change in just one variable, such as the background colour, can change many pixels in the image, which can lead to drastic changes in the agent's latent representation of the image, causing the learned policy to fail. To learn more robust representations, we introduce TEmporal Disentanglement (TED), a self-supervised auxiliary task that leads to disentangled image representations exploiting the sequential nature of RL observations. We find empirically that RL algorithms utilising TED as an auxiliary task adapt more quickly to changes in environment variables with continued training compared to state-of-the-art representation learning methods. Since TED enforces a disentangled structure of the representation, we also find that policies trained with TED generalise better to unseen values of variables irrelevant to the task (e.g. background colour) as well as unseen values of variables that affect the optimal policy (e.g. goal positions).
@inproceedings{dunion2023ted,
title={Temporal Disentanglement of Representations for Improved Generalisation in Reinforcement Learning},
author={Mhairi Dunion and Trevor McInroe and Kevin Sebastian Luck and Josiah Hanna and Stefano V. Albrecht},
booktitle={International Conference on Learning Representations (ICLR)},
year={2023}
}
Yi Ren, Shangmin Guo, Wonho Bae, Danica J. Sutherland
How to Prepare Your Task Head for Finetuning
International Conference on Learning Representations, 2023
Abstract | BibTex | arXiv
ICLRdeep-learningtransfer-learning
Abstract:
In the era of deep learning, transferring information from a pretrained network to a downstream task by finetuning has many benefits. The choice of task head plays an important role in fine-tuning, as the pretrained and downstream tasks are usually different. Although there exist many different designs for finetuning, a full understanding of when and why these algorithms work has been elusive. We analyze how the choice of task head controls feature adaptation and hence influences the downstream performance. By decomposing the feature's learning dynamics, we find the key aspect is the training accuracy and loss at the beginning of finetuning, which determines the "energy" available for the feature's adaptation. We identify a significant trend in the effect of changes in this initial energy on the resulting features after finetuning. Specifically, as the energy increases, the Euclidean and cosine distances between the resulting and original features increase, while their dot product (and the resulting features’ norm) first increases and then decreases. Inspired by this, we give several practical principles that lead to better downstream performance. We analytically prove this trend in an overparamterized linear setting and verify its applicability to different experimental settings.
@inproceedings{ ren2023how,
title={How to Prepare Your Task Head for Finetuning},
author={Yi Ren and Shangmin Guo and Wonho Bae and Danica J. Sutherland},
booktitle={International Conference on Learning Representations (ICLR)},
year={2023},
url={https://openreview.net/forum?id=gVOXZproe-e}
}
2022
Shangmin Guo, Yi Ren, Kory Mathewson, Simon Kirby, Stefano V. Albrecht, Kenny Smith
Expressivity of Emergent Languages is a Trade-off between Contextual Complexity and Unpredictability
International Conference on Learning Representations, 2022
Abstract | BibTex | arXiv | Code
ICLRmulti-agent-rlemergent-communication
Abstract:
Researchers are using deep learning models to explore the emergence of language in various language games, where simulated agents interact and develop an emergent language to solve a task. We focus on the factors which determine the expressivity of emergent languages, which reflects the amount of information about input spaces those languages are capable of encoding. We measure the expressivity of emergent languages based on their generalisation performance across different games, and demonstrate that the expressivity of emergent languages is a trade-off between the complexity and unpredictability of the context those languages are used in. Another novel contribution of this work is the discovery of message type collapse. We also show that using the contrastive loss proposed by Chen et al. (2020) can alleviate this problem, compared with the standard referential loss used by the existing works.
@inproceedings{guo2022expressivity,
title={Expressivity of Emergent Languages is a Trade-off between Contextual Complexity and Unpredictability},
author={Shangmin Guo and Yi Ren and Kory Mathewson and Simon Kirby and Stefano V. Albrecht and Kenny Smith},
booktitle={International Conference on Learning Representations (ICLR)},
year={2022}
}