Publications
For news about publications, follow us on X: 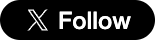
Click on any author names or tags to filter publications.
All topic tags:
surveydeep-rlmulti-agent-rlagent-modellingad-hoc-teamworkautonomous-drivinggoal-recognitionexplainable-aicausalgeneralisationsecurityemergent-communicationiterated-learningintrinsic-rewardsimulatorstate-estimationdeep-learningtransfer-learning
Selected tags (click to remove):
ICLRKenny-Smith
2024
Shangmin Guo, Yi Ren, Stefano V. Albrecht, Kenny Smith
lpNTK: Better Generalisation with Less Data via Sample Interaction During Learning
International Conference on Learning Representations, 2024
Abstract | BibTex | arXiv | Code
ICLRdeep-learning
Abstract:
Although much research has been done on proposing new models or loss functions to improve the generalisation of artificial neural networks (ANNs), less attention has been directed to the impact of the training data on generalisation. In this work, we start from approximating the interaction between samples, i.e. how learning one sample would modify the model's prediction on other samples. Through analysing the terms involved in weight updates in supervised learning, we find that labels influence the interaction between samples. Therefore, we propose the labelled pseudo Neural Tangent Kernel (lpNTK) which takes label information into consideration when measuring the interactions between samples. We first prove that lpNTK asymptotically converges to the empirical neural tangent kernel in terms of the Frobenius norm under certain assumptions. Secondly, we illustrate how lpNTK helps to understand learning phenomena identified in previous work, specifically the learning difficulty of samples and forgetting events during learning. Moreover, we also show that using lpNTK to identify and remove poisoning training samples does not hurt the generalisation performance of ANNs.
@inproceedings{guo2024lpntk,
title={Sample Relationship from Learning Dynamics Matters for Generalisation},
author={Shangmin Guo and Yi Ren and Stefano V. Albrecht and Kenny Smith},
booktitle={12th International Conference on Learning Representations},
year={2024},
url={https://openreview.net/forum?id=8Ju0VmvMCW}
}
2022
Shangmin Guo, Yi Ren, Kory Mathewson, Simon Kirby, Stefano V. Albrecht, Kenny Smith
Expressivity of Emergent Languages is a Trade-off between Contextual Complexity and Unpredictability
International Conference on Learning Representations, 2022
Abstract | BibTex | arXiv | Code
ICLRmulti-agent-rlemergent-communication
Abstract:
Researchers are using deep learning models to explore the emergence of language in various language games, where simulated agents interact and develop an emergent language to solve a task. We focus on the factors which determine the expressivity of emergent languages, which reflects the amount of information about input spaces those languages are capable of encoding. We measure the expressivity of emergent languages based on their generalisation performance across different games, and demonstrate that the expressivity of emergent languages is a trade-off between the complexity and unpredictability of the context those languages are used in. Another novel contribution of this work is the discovery of message type collapse. We also show that using the contrastive loss proposed by Chen et al. (2020) can alleviate this problem, compared with the standard referential loss used by the existing works.
@inproceedings{guo2022expressivity,
title={Expressivity of Emergent Languages is a Trade-off between Contextual Complexity and Unpredictability},
author={Shangmin Guo and Yi Ren and Kory Mathewson and Simon Kirby and Stefano V. Albrecht and Kenny Smith},
booktitle={International Conference on Learning Representations (ICLR)},
year={2022}
}