Publications
For news about publications, follow us on X: 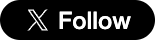
Click on any author names or tags to filter publications.
All topic tags:
surveydeep-rlmulti-agent-rlagent-modellingad-hoc-teamworkautonomous-drivinggoal-recognitionexplainable-aicausalgeneralisationsecurityemergent-communicationiterated-learningintrinsic-rewardsimulatorstate-estimationdeep-learningtransfer-learning
Selected tags (click to remove):
Kale-ab-TesseraArrasy-Rahmanmulti-agent-rl
2025
Kale-ab Tessera, Arrasy Rahman, Stefano V. Albrecht
HyperMARL: Adaptive Hypernetworks for Multi-Agent RL
arXiv:2412.04233, 2025
Abstract | BibTex | arXiv
multi-agent-rl
Abstract:
Adaptability is critical in cooperative multi-agent reinforcement learning (MARL), where agents must learn specialised or homogeneous behaviours for diverse tasks. While parameter sharing methods are sample-efficient, they often encounter gradient interference among agents, limiting their behavioural diversity. Conversely, non-parameter sharing approaches enable specialisation, but are computationally demanding and sample-inefficient. To address these issues, we propose HyperMARL, a parameter sharing approach that uses hypernetworks to dynamically generate agent-specific actor and critic parameters, without altering the learning objective or requiring preset diversity levels. By decoupling observation- and agent-conditioned gradients, HyperMARL empirically reduces policy gradient variance and facilitates specialisation within FuPS, suggesting it can mitigate cross-agent interference. Across multiple MARL benchmarks involving up to twenty agents -- and requiring homogeneous, heterogeneous, or mixed behaviours -- HyperMARL consistently performs competitively with fully shared, non-parameter-sharing, and diversity-promoting baselines, all while preserving a behavioural diversity level comparable to non-parameter sharing. These findings establish hypernetworks as a versatile approach for MARL across diverse environments.
@misc{tessera2025hyper,
title={{HyperMARL}: Adaptive Hypernetworks for Multi-Agent RL},
author={Kale-ab Tessera and Arrasy Rahman and Stefano V. Albrecht},
year={2025},
eprint={2412.04233},
archivePrefix={arXiv}
}
2024
Kale-ab Tessera, Arrasy Rahman, Stefano V. Albrecht
HyperMARL: Adaptive Hypernetworks for Multi-Agent RL
arXiv:2412.04233, 2024
Abstract | BibTex | arXiv
multi-agent-rl
Abstract:
Balancing individual specialisation and shared behaviours is a critical challenge in multi-agent reinforcement learning (MARL). Existing methods typically focus on encouraging diversity or leveraging shared representations. Full parameter sharing (FuPS) improves sample efficiency but struggles to learn diverse behaviours when required, while no parameter sharing (NoPS) enables diversity but is computationally expensive and sample inefficient. To address these challenges, we introduce HyperMARL, a novel approach using hypernetworks to balance efficiency and specialisation. HyperMARL generates agent-specific actor and critic parameters, enabling agents to adaptively exhibit diverse or homogeneous behaviours as needed, without modifying the learning objective or requiring prior knowledge of the optimal diversity. Furthermore, HyperMARL decouples agent-specific and state-based gradients, which empirically correlates with reduced policy gradient variance, potentially offering insights into its ability to capture diverse behaviours. Across MARL benchmarks requiring homogeneous, heterogeneous, or mixed behaviours, HyperMARL consistently matches or outperforms FuPS, NoPS, and diversity-focused methods, achieving NoPS-level diversity with a shared architecture. These results highlight the potential of hypernetworks as a versatile approach to the trade-off between specialisation and shared behaviours in MARL.
@misc{tessera2024hyper,
title={{HyperMARL}: Adaptive Hypernetworks for Multi-Agent RL},
author={Kale-ab Tessera and Arrasy Rahman and Stefano V. Albrecht},
year={2024},
eprint={2412.04233},
archivePrefix={arXiv}
}