Publications
For news about publications, follow us on X: 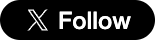
Click on any author names or tags to filter publications.
All topic tags:
surveydeep-rlmulti-agent-rlagent-modellingad-hoc-teamworkautonomous-drivinggoal-recognitionexplainable-aicausalgeneralisationsecurityemergent-communicationiterated-learningintrinsic-rewardsimulatorstate-estimationdeep-learningtransfer-learning
Selected tags (click to remove):
Lingxin-Kong
2025
Cheng Wang, Lingxin Kong, Massimiliano Tamborski, Stefano V. Albrecht
HAD-Gen: Human-like and Diverse Driving Behavior Modeling for Controllable Scenario Generation
arXiv:2503.15049, 2025
Abstract | BibTex | arXiv
autonomous-drivingagent-modelling
Abstract:
Simulation-based testing has emerged as an essential tool for verifying and validating autonomous vehicles (AVs). However, contemporary methodologies, such as deterministic and imitation learning-based driver models, struggle to capture the variability of human-like driving behavior. Given these challenges, we propose HAD-Gen, a general framework for realistic traffic scenario generation that simulates diverse human-like driving behaviors. The framework first clusters the vehicle trajectory data into different driving styles according to safety features. It then employs maximum entropy inverse reinforcement learning on each of the clusters to learn the reward function corresponding to each driving style. Using these reward functions, the method integrates offline reinforcement learning pre-training and multi-agent reinforcement learning algorithms to obtain general and robust driving policies. Multi-perspective simulation results show that our proposed scenario generation framework can simulate diverse, human-like driving behaviors with strong generalization capability. The proposed framework achieves a 90.96% goal-reaching rate, an off-road rate of 2.08%, and a collision rate of 6.91% in the generalization test, outperforming prior approaches by over 20% in goal-reaching performance.
@misc{tessera2025hyper,
title={{HAD-Gen}: Human-like and Diverse Driving Behavior Modeling for Controllable Scenario Generation},
author={Cheng Wang and Lingxin Kong and Massimiliano Tamborski and Stefano V. Albrecht},
year={2025},
eprint={503.15049},
archivePrefix={arXiv}
}