Publications
For news about publications, follow us on X: 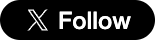
Click on any author names or tags to filter publications.
All topic tags:
surveydeep-rlmulti-agent-rlagent-modellingad-hoc-teamworkautonomous-drivinggoal-recognitionexplainable-aicausalgeneralisationsecurityemergent-communicationiterated-learningintrinsic-rewardsimulatorstate-estimationdeep-learningtransfer-learning
Selected tags (click to remove):
Trevor-McInroeICLR
2025
Samuel Garcin, Trevor McInroe, Pablo Samuel Castro, Christopher G. Lucas, David Abel, Prakash Panangaden, Stefano V. Albrecht
Studying the Interplay Between the Actor and Critic Representations in Reinforcement Learning
International Conference on Learning Representations, 2025
Abstract | BibTex | Paper | Code
ICLRdeep-rlgeneralisation
Abstract:
Extracting relevant information from a stream of high-dimensional observations is a central challenge for deep reinforcement learning agents. Actor-critic algorithms add further complexity to this challenge, as it is often unclear whether the same information will be relevant to both the actor and the critic. To this end, we here explore the principles that underlie effective representations for an actor and for a critic. We focus our study on understanding whether an actor and a critic will benefit from a decoupled, rather than shared, representation. Our primary finding is that when decoupled, the representations for the actor and critic systematically specialise in extracting different types of information from the environment---the actor's representation tends to focus on action-relevant information, while the critic's representation specialises in encoding value and dynamics information. Finally, we demonstrate how these insights help select representation learning objectives that play into the actor's and critic's respective knowledge specialisations, and improve performance in terms of agent returns.
@inproceedings{garcin2025acrep,
title={Studying the Interplay Between the Actor and Critic Representations in Reinforcement Learning},
author={Samuel Garcin and Trevor McInroe and Pablo Samuel Castro and Christopher G. Lucas and David Abel and Prakash Panangaden and Stefano V. Albrecht},
booktitle={13th International Conference on Learning Representations},
year={2025}
}
2023
Mhairi Dunion, Trevor McInroe, Kevin Sebastian Luck, Josiah Hanna, Stefano V. Albrecht
Temporal Disentanglement of Representations for Improved Generalisation in Reinforcement Learning
International Conference on Learning Representations, 2023
Abstract | BibTex | arXiv | Code
ICLRdeep-rlgeneralisationcausal
Abstract:
Reinforcement Learning (RL) agents are often unable to generalise well to environment variations in the state space that were not observed during training. This issue is especially problematic for image-based RL, where a change in just one variable, such as the background colour, can change many pixels in the image, which can lead to drastic changes in the agent's latent representation of the image, causing the learned policy to fail. To learn more robust representations, we introduce TEmporal Disentanglement (TED), a self-supervised auxiliary task that leads to disentangled image representations exploiting the sequential nature of RL observations. We find empirically that RL algorithms utilising TED as an auxiliary task adapt more quickly to changes in environment variables with continued training compared to state-of-the-art representation learning methods. Since TED enforces a disentangled structure of the representation, we also find that policies trained with TED generalise better to unseen values of variables irrelevant to the task (e.g. background colour) as well as unseen values of variables that affect the optimal policy (e.g. goal positions).
@inproceedings{dunion2023ted,
title={Temporal Disentanglement of Representations for Improved Generalisation in Reinforcement Learning},
author={Mhairi Dunion and Trevor McInroe and Kevin Sebastian Luck and Josiah Hanna and Stefano V. Albrecht},
booktitle={International Conference on Learning Representations (ICLR)},
year={2023}
}