Publications
For news about publications, follow us on X: 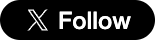
Click on any author names or tags to filter publications.
All topic tags:
surveydeep-rlmulti-agent-rlagent-modellingad-hoc-teamworkautonomous-drivinggoal-recognitionexplainable-aicausalgeneralisationsecurityemergent-communicationiterated-learningintrinsic-rewardsimulatorstate-estimationdeep-learningtransfer-learning
Selected tags (click to remove):
simulator
2024
Aleksandar Krnjaic, Raul D. Steleac, Jonathan D. Thomas, Georgios Papoudakis, Lukas Schäfer, Andrew Wing Keung To, Kuan-Ho Lao, Murat Cubuktepe, Matthew Haley, Peter Börsting, Stefano V. Albrecht
Scalable Multi-Agent Reinforcement Learning for Warehouse Logistics with Robotic and Human Co-Workers
IEEE/RSJ International Conference on Intelligent Robots and Systems, 2024
Abstract | BibTex | arXiv | Website
IROSmulti-agent-rlsimulator
Abstract:
We envision a warehouse in which dozens of mobile robots and human pickers work together to collect and deliver items within the warehouse. The fundamental problem we tackle, called the order-picking problem, is how these worker agents must coordinate their movement and actions in the warehouse to maximise performance (e.g. order throughput). Established industry methods using heuristic approaches require large engineering efforts to optimise for innately variable warehouse configurations. In contrast, multi-agent reinforcement learning (MARL) can be flexibly applied to diverse warehouse configurations (e.g. size, layout, number/types of workers, item replenishment frequency), as the agents learn through experience how to optimally cooperate with one another. We develop hierarchical MARL algorithms in which a manager assigns goals to worker agents, and the policies of the manager and workers are co-trained toward maximising a global objective (e.g. pick rate). Our hierarchical algorithms achieve significant gains in sample efficiency and overall pick rates over baseline MARL algorithms in diverse warehouse configurations, and substantially outperform two established industry heuristics for order-picking systems
@inproceedings{krnjaic2024scalable,
title={Scalable Multi-Agent Reinforcement Learning for Warehouse Logistics with Robotic and Human Co-Workers},
author={Aleksandar Krnjaic and Raul D. Steleac and Jonathan D. Thomas and Georgios Papoudakis and Lukas Sch\"afer and Andrew Wing Keung To and Kuan-Ho Lao and Murat Cubuktepe and Matthew Haley and Peter B\"orsting and Stefano V. Albrecht},
booktitle={IEEE/RSJ International Conference on Intelligent Robots and Systems},
year={2023}
}
2023
Giuseppe Vecchio, Simone Palazzo, Dario C Guastella, Riccardo E. Sarpietro, Ignacio Carlucho, Stefano V. Albrecht, Giovanni Muscato, Concetto Spampinato
MIDGARD: A Simulation Platform for Autonomous Navigation in Unstructured Environments
RSS Workshop on Multi-Agent Planning and Navigation in Challenging Environments, 2023
Abstract | BibTex | arXiv
RSSsimulatordeep-rl
Abstract:
We present MIDGARD, an open-source simulation platform for autonomous robot navigation in outdoor unstructured environments. MIDGARD is designed to enable the training of autonomous agents (e.g., unmanned ground vehicles) in photorealistic 3D environments, and to support the generalization skills of learning-based agents through the variability in training scenarios. MIDGARD's main features include a configurable, extensible, and difficulty-driven procedural landscape generation pipeline, with fast and photorealistic scene rendering based on Unreal Engine. Additionally, MIDGARD has built-in support for OpenAI Gym, a programming interface for feature extension (e.g., integrating new types of sensors, customizing exposing internal simulation variables), and a variety of simulated agent sensors (e.g., RGB, depth and instance/semantic segmentation). We evaluate MIDGARD's capabilities as a benchmarking tool for robot navigation utilizing a set of state-of-the-art reinforcement learning algorithms. The results demonstrate MIDGARD's suitability as a simulation and training environment, as well as the effectiveness of our procedural generation approach in controlling scene difficulty, which directly reflects on accuracy metrics.
@inproceedings{vecchio2022midgard,
title={MIDGARD: A Simulation Platform for Autonomous Navigation in Unstructured Environments},
author={Vecchio, Giuseppe and Palazzo, Simone and Guastella, Dario C and Sarpietro, Riccardo E. and Carlucho, Ignacio and Albrecht, Stefano V. and Muscato, Giovanni and Spampinato, Concetto},
booktitle={RSS 2023 Workshop on Multi-Agent Planning and Navigation in Challenging Environments},
year={2023}
}
Aleksandar Krnjaic, Raul D. Steleac, Jonathan D. Thomas, Georgios Papoudakis, Lukas Schäfer, Andrew Wing Keung To, Kuan-Ho Lao, Murat Cubuktepe, Matthew Haley, Peter Börsting, Stefano V. Albrecht
Scalable Multi-Agent Reinforcement Learning for Warehouse Logistics with Robotic and Human Co-Workers
arXiv:2212.11498, 2023
Abstract | BibTex | arXiv | Website
multi-agent-rlsimulator
Abstract:
We envision a warehouse in which dozens of mobile robots and human pickers work together to collect and deliver items within the warehouse. The fundamental problem we tackle, called the order-picking problem, is how these worker agents must coordinate their movement and actions in the warehouse to maximise performance (e.g. order throughput). Established industry methods using heuristic approaches require large engineering efforts to optimise for innately variable warehouse configurations. In contrast, multi-agent reinforcement learning (MARL) can be flexibly applied to diverse warehouse configurations (e.g. size, layout, number/types of workers, item replenishment frequency), as the agents learn through experience how to optimally cooperate with one another. We develop hierarchical MARL algorithms in which a manager assigns goals to worker agents, and the policies of the manager and workers are co-trained toward maximising a global objective (e.g. pick rate). Our hierarchical algorithms achieve significant gains in sample efficiency and overall pick rates over baseline MARL algorithms in diverse warehouse configurations, and substantially outperform two established industry heuristics for order-picking systems
@misc{krnjaic2023scalable,
title={Scalable Multi-Agent Reinforcement Learning for Warehouse Logistics with Robotic and Human Co-Workers},
author={Aleksandar Krnjaic and Raul D. Steleac and Jonathan D. Thomas and Georgios Papoudakis and Lukas Sch\"afer and Andrew Wing Keung To and Kuan-Ho Lao and Murat Cubuktepe and Matthew Haley and Peter B\"orsting and Stefano V. Albrecht},
year={2023},
eprint={2212.11498},
archivePrefix={arXiv},
primaryClass={cs.LG}
}
2022
Giuseppe Vecchio, Simone Palazzo, Dario C Guastella, Ignacio Carlucho, Stefano V. Albrecht, Giovanni Muscato, Concetto Spampinato
MIDGARD: A Simulation Platform for Autonomous Navigation in Unstructured Environments
ICRA Workshop on Releasing Robots into the Wild: Simulations, Benchmarks, and Deployment, 2022
Abstract | BibTex | arXiv
ICRAdeep-rlsimulator
Abstract:
We present MIDGARD, an open source simulation platform for autonomous robot navigation in unstructured outdoor environments. We specifically design MIDGARD to enable training of autonomous agents (e.g., unmanned ground vehicles) in photorealistic 3D environments, and to support the generalization skills of learning-based agents by means of diverse and variable training scenarios. MIDGARD differs from other major simulation platforms in that it proposes a highly configurable procedural landscape generation pipeline, which enables autonomous agents to be trained in diverse scenarios while reducing the efforts and costs needed to create digital content from scratch.
@misc{Vecchio2022MIDGARD,
title={MIDGARD: A Simulation Platform for Autonomous Navigation in Unstructured Environments},
author={Giuseppe Vecchio, Simone Palazzo, Dario C Guastella, Ignacio Carlucho, Stefano V. Albrecht, Giovanni Muscato, Concetto Spampinato},
year={2022},
eprint={2205.08389},
archivePrefix={arXiv},
primaryClass={cs.MA}
}